How do you know if data is missing randomly?
Índice
- How do you know if data is missing randomly?
- Is data missing at random?
- What is data missing completely at random?
- How do you check for missing data?
- How do you handle missing Mnar data?
- How do you deal with missing random data?
- What percentage of missing data is acceptable?
- When should you delete missing data?
- How much missing data is too much?
- What to do when data are missing not at random?
- Which is ignorable missing completely or missing at random?
- What does it mean when data is missing?
- What does missing completely at random MCAR mean?
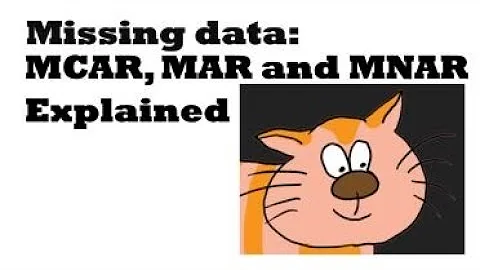
How do you know if data is missing randomly?
1. The only true way to distinguish between MNAR and Missing at Random is to measure the missing data. In other words, you need to know the values of the missing data to determine if it is MNAR. It is common practice for a surveyor to follow up with phone calls to the non-respondents and get the key information.
Is data missing at random?
Missing Completely at Random, MCAR, means there is no relationship between the missingness of the data and any values, observed or missing. Those missing data points are a random subset of the data. There is nothing systematic going on that makes some data more likely to be missing than others.
What is data missing completely at random?
When we say data are missing completely at random, we mean that the missingness is nothing to do with the person being studied. ... When we say data are missing at random, we mean that the missingness is to do with the person but can be predicted from other information about the person.
How do you check for missing data?
These are the five steps to ensuring missing data are correctly identified and appropriately dealt with:
- Ensure your data are coded correctly.
- Identify missing values within each variable.
- Look for patterns of missingness.
- Check for associations between missing and observed data.
- Decide how to handle missing data.
How do you handle missing Mnar data?
Techniques for Handling the Missing Data
- Listwise or case deletion. ...
- Pairwise deletion. ...
- Mean substitution. ...
- Regression imputation. ...
- Last observation carried forward. ...
- Maximum likelihood. ...
- Expectation-Maximization. ...
- Multiple imputation.
How do you deal with missing random data?
Best techniques to handle missing data
- Use deletion methods to eliminate missing data. The deletion methods only work for certain datasets where participants have missing fields. ...
- Use regression analysis to systematically eliminate data. ...
- Data scientists can use data imputation techniques.
What percentage of missing data is acceptable?
Proportion of missing data Yet, there is no established cutoff from the literature regarding an acceptable percentage of missing data in a data set for valid statistical inferences. For example, Schafer ( 1999 ) asserted that a missing rate of 5% or less is inconsequential.
When should you delete missing data?
If data is missing for more than 60% of the observations, it may be wise to discard it if the variable is insignificant.
How much missing data is too much?
Statistical guidance articles have stated that bias is likely in analyses with more than 10% missingness and that if more than 40% data are missing in important variables then results should only be considered as hypothesis generating [18], [19].
What to do when data are missing not at random?
- It’s possible to do multiple imputation or maximum likelihood when data are missing not at random (MNAR), but to do that, you first need to specify a model for the missing data mechanism—that is, a model of how missingness depends on both observed and unobserved quantities.
Which is ignorable missing completely or missing at random?
- “Missing Completely at Random” and “Missing at Random” are both considered ‘ignorable’ because we don’t have to include any information about the missing data itself when we deal with the missing data. Multiple imputation and Maximum Likelihood assume the data are at least missing at random.
What does it mean when data is missing?
- Roughly speaking, this means that the probability that data are missing on a particular variable does not depend on the value of that variable, after adjusting for observed variables. This assumption would be violated, for example, if people with high income were less likely to report their income.
What does missing completely at random MCAR mean?
- Missing Completely at Random, MCAR, means there is no relationship between the missingness of the data and any values, observed or missing. Those missing data points are a random subset of the data.